synthetic environment approach
The added value of the simulated data in the efficiency of image recognition training process
Thanks to the latest improvements in machine and deep learning algorithm, intelligent imaged recognition systems have reached a new step in their performance level. However, these technologies have reached their limitation as they greatly rely on the amount of available source data to feed the algorithm and “teach it the right solution”. Synthetic images could appear as good way to tackle this scarcity of real data as long as their realism can be guaranteed.
A recent study made by IBM with OKTAL-SE simulation tools shows clearly the added value of the simulated data in the efficiency of image recognition training process (here).
With the synthetic environment approach, the user has a complete control of the scene parameters so he can define the appropriate sampling of each of them to cover a wide range of situation.
See our White Paper for more information.
Aside the validity of the generated images, this kind of approach presents a set of key advantages:
- To evaluate the efficiency of a detection algorithm, it is relevant to know the solution in advance. In a simulated image, the object to detect is known, as it has been created and positioned in the simulated environment.
- Thanks to our Synthetic Environment, we can change parameters such as: weather conditions, time of day, season, visibility, atmosphere type, clouds, etc
- We can generate typical images: rural area, urban, industrial, desert area, maritime environment, mountains, etc.
- Thanks to the virtual sensor positioning, we can vary direction, distance and field of view of the sensor.
- We can generate different types of sensor images: electro-optics (visible band or any infrared bands: SWIR, MWIR or LWIR), RADAR (band X to W)
- We can generate different sensor effects: blur, jittering, distortion, white balance
Image provider
Services
OKTAL-SE can provide you with image dataset of a given scenario (target, background, sensor positioning and parameters)
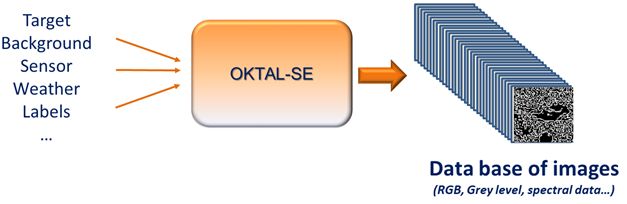
Should the user want to manage the image generation process then OKTAL-SE provides simulation tools covering visible (RGB), Spectral EO (visible and infrared) and radar domains. See this short description of a dedicated package for massive SAR image generation.
Thanks to its multi-sensor and physics based simulation workbench, our synthetic spectral data can be used in fusion algorithm (see a recent study of Recognition application using Deep Learning algorithm and data fusion: here).
For more information on Deep Learning services, please contact: sales@oktal-se.fr.